Uncategorized
A-levels, AI and a really big mess – lessons from the Ofqual algorithm
Article by:Victoria Hourigan

Related Articles
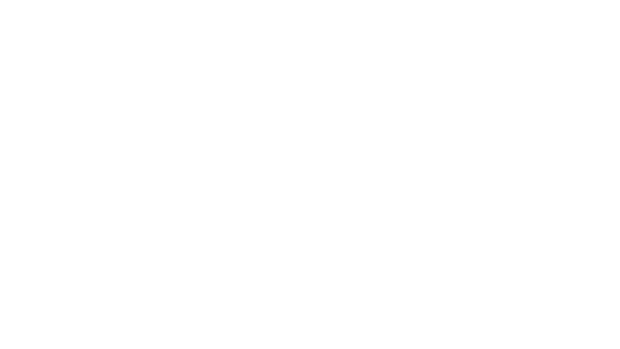
Unbabel selects CommsCo to take its AI-powered translation message to the media
Article by:Ryan Seller